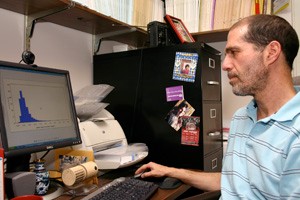
Crunching Numbers for More Accurate Blood Tests
Most patients who receive blood tests dont see the report that comes back from the lab describing the healthy or unhealthy levels of chemical analytes in their blood. But if they did, they would notice a range of numbers for each tested analyte that a healthy individual should fall into.
If the patients numbers reside within the range, they may get a green light from the physician and be considered healthy. Should the patient fall out of the normal range, however, a red flag may be raised for the physician to further investigate.
But these ranges arent static. What determines a normal range for these chemical analytes changes not only across countries or states, but laboratories as well. Theoretically, then, a patient can get the same blood test analyzed by two separate labs and get conflicting results.
The reason is because laboratories must determine their own normal rangesor reference intervals as statisticians and clinical chemists call themto best fit the population it serves. While your triglyceride count resides within normal limits in Cincinnati, it may be red-flagged in Beijing.
So how does a laboratory determine these intervals exactly?
Paul Horn, a statistics professor in McMickens Department of Mathematical Sciences, has dedicated much of his research to the topic. In fact, the work he does on reference intervals is influential enough to have an effect on any individual in the worldall due to his collaboration on the recent guidelines set forth by the Clinical and Laboratory Standards Institute (CLSI).
It was back in 1994 when Horn began working with College of Medicine Professor Amadeo Pesce. When his statistical interests overlapped Pesces research in clinical chemistry, they started to collaborate on research needed for reference intervals.
Professor Pesce was interested in reference intervals and I was interested in quantileor percentileestimation, and it turns out that they are the same problem. The basic idea is that we want to figure out what the lower and upper cut offs are for the healthy populations.
The two even co-wrote a book on reference intervals that was published in 2005.
Today, Horn is considered among colleagues as an expert on the subject, confirmed by his invitation a few years ago to join an advisory board as the sole pure statistician of the CLSI. The board worked together on an updated guideline that sets reference interval standards for laboratories worldwide.
Defining, Establishing, and Verifying Reference Intervals in the Clinical Laboratory; Approved GuidelineThird Edition was released in December after years of collaboration.
Horns work with the institute involves describing the methods he uses for constructing reference intervals that are robust, meaning they are not as sensitive to outliers in the data, they use the data efficiently, and work well even when based on a relatively small sample.
When a laboratory tries to determine the reference intervals of a healthy population, it aims to do so with a sample size of 120 individuals. This sample size allows for the upper and lower 2.5 percentiles of the distribution to be accurately estimated, thus yielding a 95 percent prediction interval that demonstrates the normal range the chemical analytes should fall into.
The question is what if you only have 80 people in your sample, Horn said. It can get very tricky because a lot of times, these measurements can be gender, age or race specific, Horn explained. Rather than only needing 120 healthy individuals, laboratories might need more specific calculations, like 120 healthy African-American males between the ages of 50 and 60.
It can be quite a large task for a laboratory.
By working on robust models, however, Horn is able to lower the sample size while not compromising the confidence interval, thus allowing for a more efficient way of determining reference intervals. While hes been able to lower the sample size significantly, CLSI still recommends having a sample size of at least 80 healthy individuals to determine accurate reference intervals.
The method Ive developed may be used now as an alternative, Horn said. The idea is that we will try to extract as much information from the data as possible without letting the outliers have too much influence.
Horn worked with the CLSI for three years on the guidelines, and felt a sense of accomplishment when he finally received a hard copy of the new edition in the mail. Yet hell be the first to admit that his work is nowhere near completion.
Like all research, there are always more questions, Horn said. You have to keep asking new questions to avoid getting tired of the same area.
The professor said hell continue to conduct his research on reference intervals, but plans to shift gears from robust models to focus on other statistical problems, involving a unified approach to data transformation and outlier detection.
Related Stories
Pediatrician celebrates 30th UC College of Medicine reunion with...
April 3, 2025
There’s a vital thread woven into the fabric of Chris Peltier’s life. It’s black and red, and it knits all things Bearcat together into a life that was transformed by the University of Cincinnati and the UC College of Medicine.
Hands-on experiences, real-world impact
April 3, 2025
University of Cincinnati second-year medical sciences student Jay Patel is pursuing his passions as founder of the Dahyabhai Foundation, a cancer researcher and a Portman Fellow.
UC Journalism to host Hall of Fame, Young Alumni Awards
Event: April 24, 2025 6:00 PM
The College of Arts and Sciences’ journalism department will host the Hall of Fame and Young Alumni Awards to celebrate the achievements and accomplishments of its graduates. Induction into the UC Journalism Hall of Fame is a special honor reserved for UC alumni who have excelled in the profession of journalism and media, or individuals who have made a significant contribution to journalism at UC.